MTA and MMM are powerful when used separately, but when used together, they can be transformative for marketers
Modern customer journeys are long, with myriad touchpoints between initial awareness and the ultimate decision. One shopper might end up on your website by way of an organic search but won’t make a purchase until reading a recommendation on a social platform. Another might come to you via email, return after conducting a little research on a referral site, visit again following a click on a banner ad and then finally buy your product after reading a recommendation on that same social platform.
Download our Premium Resource – Online Customer Acquisition Plan guide
The guide features a structured approach to creating an online acquisition plan by reviewing current performance; defining the right KPIs to control acquisition; creating a media and content engagement strategy; creating a zero-based budget model and reviewing attribution and tracking.
Access the Online customer acquisition guide
While it would be unfair to say that the only channel influencing the purchase is social, that’s still one of the most common methods of attribution: 100% of the credit goes to the last click.
Today, marketing performance measurement is far more sophisticated than this. Many of the tools now available allow you to have a more holistic view of what’s driving your business by way of marketing mix modelling (MMM), multitouch attribution (MTA), or a combination of these two approaches.
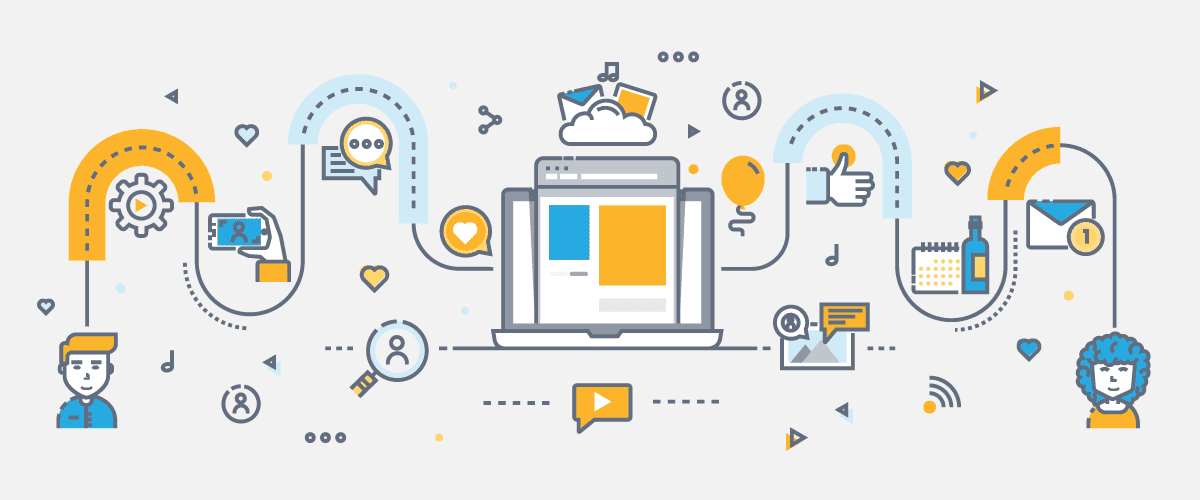
MMM and MTA: Working together in harmony
Marketing mix models are used to measure the contributions of various media investments and external drivers on business outcomes. They essentially “mix” your chosen marketing channels with historical or temporal performance data to illustrate the relationship between your activity in any one channel and the impact it might’ve had on sales — while still accounting for the impact of other factors like seasonality, weather, new product launches, or economic trends.
Let’s say, for example, you’re running a TV campaign. MMM allows you to measure the contribution of this advertising investment by comparing the sales in the periods when the ad ran versus the sales in the periods when the campaign was dark. All this while controlling for other drivers like digital media channels and variables like pricing. You’d know whether you had struck the ideal mix of marketing channels to drive the greatest ROI.
MTA, on the other hand, is designed to assign credit to the individual touchpoints that affect consumers’ path to purchase. These models are built to measure each channel’s contribution along the customer’s journey, providing a clearer picture of which investments (or combination of investments) improve key business metrics.
If you were running an omnichannel marketing campaign, an MTA model would leverage data from the paths of both buyers and non-buyers. Then, by comparing the characteristics of each path and the unique attributes of each consumer, you’d find the combination of touchpoints that increase the likelihood of conversion — or non-conversion.
In other words, MMM gives you a top-down, macro-level view of your marketing efforts across all channels, while MTA provides a bottom-up, granular view of your approach.
When you combine MMM and MTA methodologies, you arrive at a unified measurement approach, one in which multiple types of data sets, techniques, and approaches illustrate not only each channel’s impact on sales but also how the interaction between channels and non-media variables can influence buying decisions. In turn, these insights allow you to know where to invest your ad spend for the greatest incremental impact, as well as which creative, copy, and messaging will resonate best on each channel. This allows you to be more strategic and to improve tactical decisions.
Without an integrated approach, MMM and MTA models might contradict each other, leaving marketers scratching their heads or giving up in disgust. But building a unified measurement solution from the ground up ensures alignment for long-term planning and near-term optimization. It also supports more accurate forecasting for budgeting purposes.
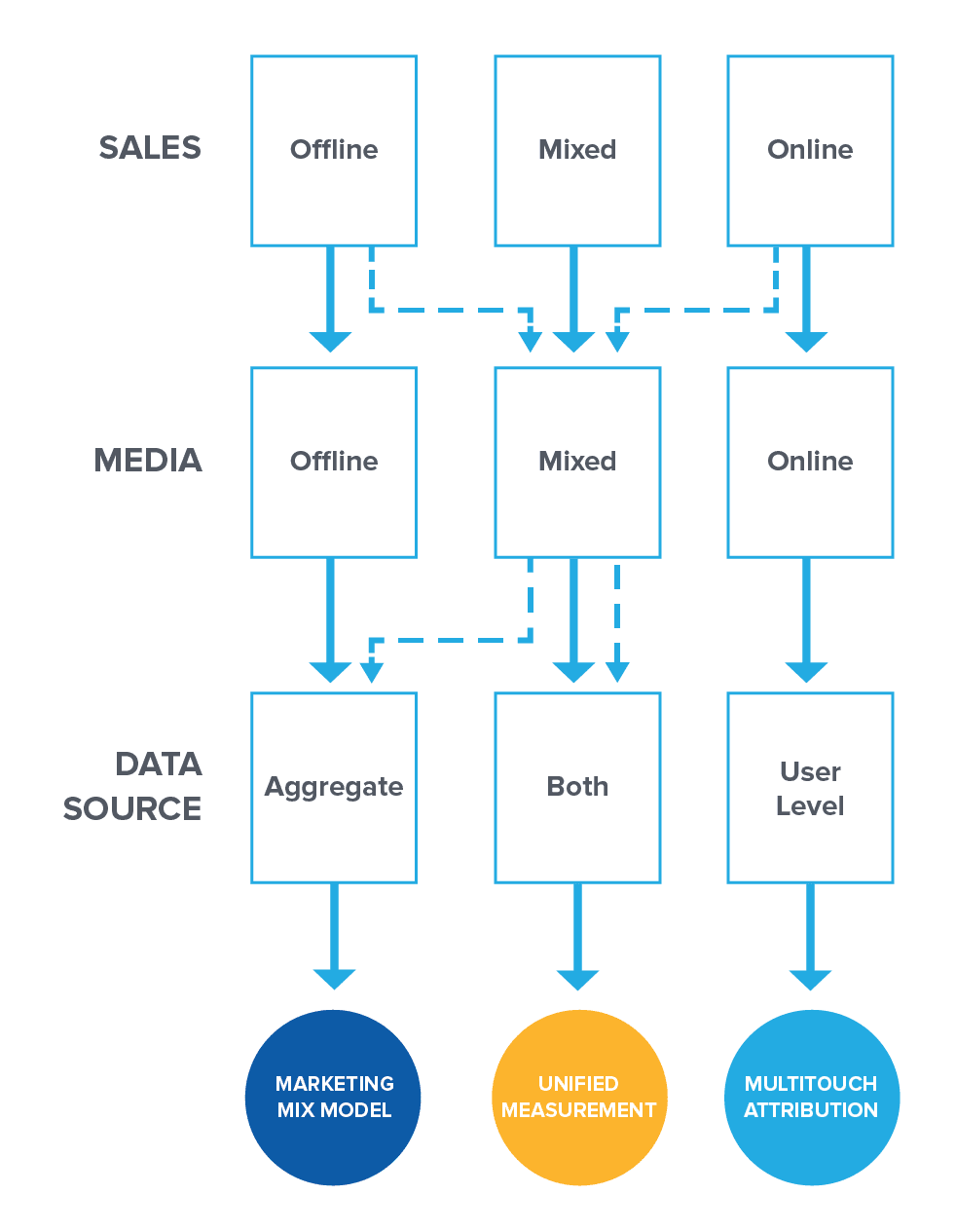
Performance enhancers
When structured correctly in a unified measurement framework, MMM and MTA complement each other. Without having a unified view, it’s easy to understand why they might contradict and cause problems for marketers. A key difference is that at the core, MMM and MTA use different measurements for evaluating ROI.
MMM has evolved over the past several years to incorporate hierarchical bayesian approaches, in which model parameters are estimated using complete data sets and information is flowing from different units of observation. A hierarchy of relationships is defined and coefficients are estimated using that hierarchy. This hierarchy creates a complex data infrastructure, which was historically hard to make sense of, but recent advances in artificial intelligence and machine learning have vastly improved capabilities with these approaches.
MTA models have also evolved, becoming increasingly complex. You can now measure differentiated effects by channel, sub-channel, campaign, tactic, creative, publisher, placement, etc. This allows for those granular insights mentioned previously. In fact, advanced machine learning has deepened the tactical effect of MTA, allowing marketers to estimate models with thousands of parameters without overfitting the data, as well as recalibrating the models with new data on a daily or weekly basis.
This then leads us to the question of the hour: How do you find the right balance between MMM and MTA for success with measurement programs? The following are usually the best places to start.
1. Take a holistic approach that uses both MTA and MMM strategies.
By now, you’ve probably gathered that MMM and MTA play different roles in planning, budgeting, executing, and measuring your marketing initiatives. You should not only use both performance measurements but also leverage a solution that integrates insights from the two in a unified approach.
Just as MTA models leverage user-level behaviour to provide more accurate insights on digital and other addressable channels, MMM models provide more strategic insights into non-media drivers of demand like economic variables or competitive activities. Unified measurement solutions that leverage MMM methodologies for strategic decision-making and MTA methodologies for granular reads on particular placements deliver more accurate, actionable recommendations for planning and optimization.
2. Use the right approach to measure incrementally for each media channel.
Not all media channels are accurately measured in marketing mix models, especially when the media channel’s volume and spend are directly driven by the interest that consumers have in the product. Take paid search, for instance. As interest in a product grows, so will its search volume and paid search spend.
To accurately measure the contribution of paid search in driving sales, one would need to include a “product interest” variable in an MMM, as that variable is driving paid search volume and sales. Unfortunately, this variable isn’t easily distilled into a data set, so it usually isn’t used in MMM performance measurement models. Without the product interest variable, the results for MMM overstate the contribution of paid search.
Enter MTA, in which user-level intent can be captured using various behavioural variables (e.g. users’ site visits, previous purchases, organic clicks, etc.) to account for product interest, providing a more accurate read on the incremental impact of paid search clicks. By including behavioural variables into the MTA models, one measures the incremental contribution of paid search and can then feed these insights into the MMM.
3. Establish communication between the two models.
To balance the recommendations of MTA and MMM methodologies in a holistic framework, an exchange of data and attribution credit must take place with some regularity. MMM’s reliance on historical data can mean systems that are overly biased toward insights from MMM do not react to fast-changing consumer media usage behaviour. Likewise, MTA’s reliance on a user-identified, often online conversion point might create a blind spot when accounting for the impact of offline drivers of demand like outdoor advertising.
When the two perspectives meld, the unified measurement model applies the insights from MMM (e.g. offline media, historical drivers, total sales, other environmental factors, etc.) to those from MTA (e.g. online media, online conversions, etc.) and vice versa, taking into account both offline and online contributions to holistically measure marketing performance.
MTA and MMM are powerful when used separately, but when used together, they can be transformative for marketers. MMM provides high-level top-down insights, while MTA gives you more granular recommendations. Using the combined power of both approaches, brands can create a unified marketing strategy with greater insight into the most effective ways to reach their customers.
JP Pereira is the chief client officer at
Conversion Logic, and he is responsible for overseeing client projects, including client and data science teams. JP has extensive experience managing account teams and analytical and consulting teams for marketing analytics, market research, and strategy consulting firms.